In the rapidly evolving landscape of manufacturing, smart factories represent the pinnacle of modern industrial innovation.
These highly automated and interconnected environments rely heavily on machinery that operates seamlessly to ensure optimal production.
However, machinery failures can disrupt operations, leading to costly downtime and maintenance expenses.
This is where artificial intelligence (AI) steps in as a game-changer. By leveraging AI, smart factories can predict and prevent common machinery failures, enhancing efficiency and reducing costs.
In this article, we explore how artificial intelligence is revolutionizing predictive maintenance and preventing 10 common machinery failures in smart factories.
Introduction
Smart factories integrate advanced technologies like the Internet of Things (IoT), machine learning, and artificial intelligence to create highly efficient and responsive manufacturing systems.
Artificial intelligence, in particular, plays a critical role in predictive maintenance by analyzing vast amounts of data to identify potential issues before they cause machinery failures.
By doing so, AI not only prevents unexpected breakdowns but also extends the lifespan of equipment and optimizes production processes.
What is Artificial Intelligence in Smart Factories?
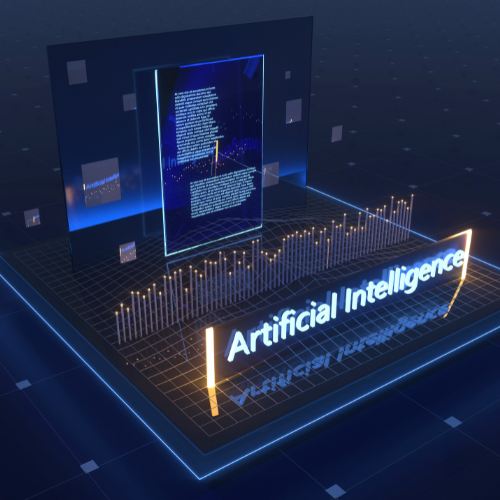
Artificial intelligence refers to the simulation of human intelligence in machines that are programmed to think and learn like humans.
In the context of smart factories, AI is used to analyze data from various sources, such as sensors and IoT devices, to make informed decisions about machinery maintenance.
AI algorithms can detect patterns and anomalies in data, predict potential failures, and recommend preventive actions. This proactive approach to maintenance is known as predictive maintenance.
The Role of Artificial Intelligence in Predictive Maintenance
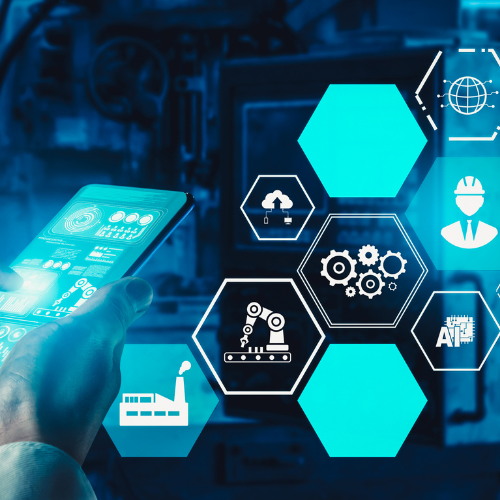
Predictive maintenance leverages artificial intelligence to monitor the condition of machinery and predict when maintenance should be performed.
This approach reduces the likelihood of unexpected failures and minimizes maintenance costs. AI achieves this by continuously analyzing data from machinery, identifying signs of wear and tear, and alerting maintenance teams to take action before a failure occurs.
Key technologies used in AI-driven predictive maintenance include machine learning algorithms, IoT sensors, and data analytics.
10 Common Machinery Failures Prevented by Artificial Intelligence
1. Bearing Failures
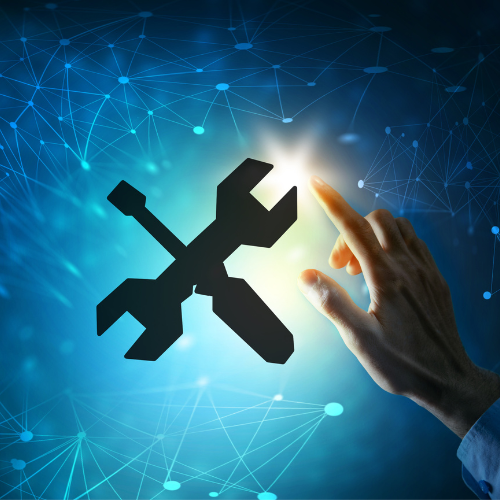
Bearings are critical components in machinery, and their failure can lead to significant operational disruptions. AI can monitor the vibration and temperature of bearings, detecting early signs of wear and tear.
By analyzing these data points, AI algorithms can predict when a bearing is likely to fail, allowing maintenance teams to replace it before it causes a breakdown.
2. Overheating Motors
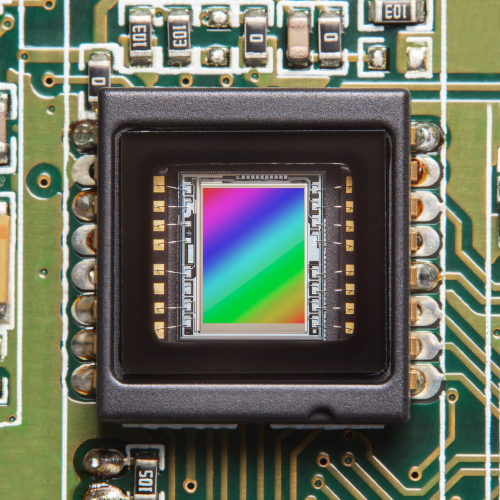
Overheating is a common issue in motors that can lead to severe damage if not addressed promptly. Artificial intelligence can continuously monitor the temperature of motors using IoT sensors.
If the temperature exceeds safe thresholds, AI systems can trigger cooling mechanisms or alert maintenance personnel to take corrective action.
3. Lubrication Issues
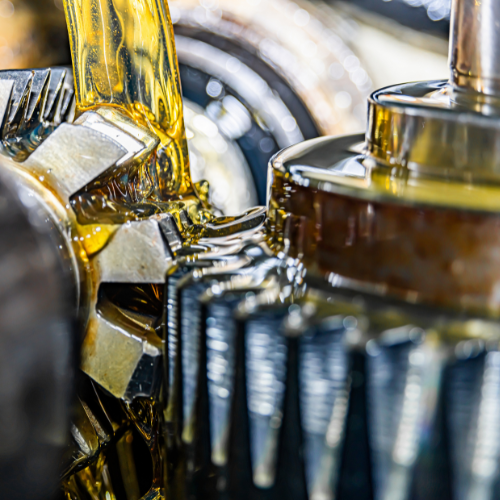
Proper lubrication is essential for the smooth operation of machinery. AI can monitor lubrication levels and viscosity, ensuring that components receive adequate lubrication.
If lubrication levels drop or the lubricant degrades, AI can schedule maintenance to replenish or replace the lubricant, preventing friction and wear.
4. Vibration Anomalies
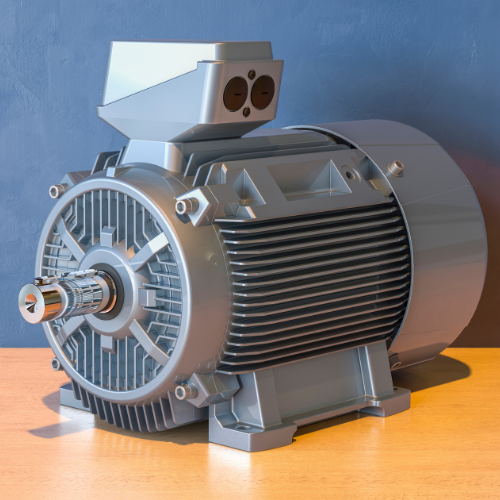
Unusual vibrations in machinery often indicate underlying problems such as misalignment or imbalances. AI-powered sensors can detect vibration anomalies and analyze their frequency and amplitude.
By identifying these patterns, AI can pinpoint the root cause of the issue and recommend maintenance to rectify it before it leads to more significant damage.
5. Electrical Failures
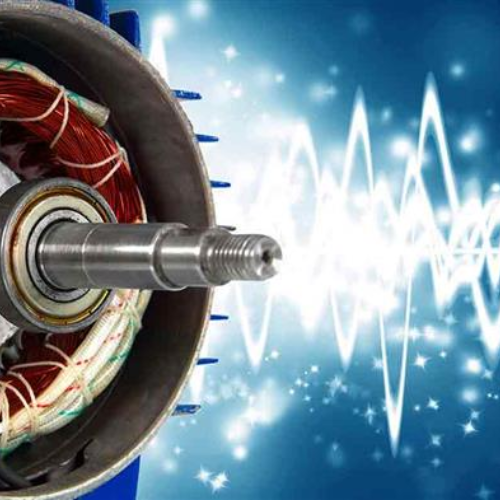
Electrical components in machinery are susceptible to failures due to various factors, including short circuits and component degradation. Artificial intelligence can monitor electrical parameters such as voltage, current, and resistance.
By analyzing these data points, AI can detect irregularities that may indicate impending electrical failures, allowing for timely intervention.
6. Wear and Tear of Belts and Chains
Belts and chains are prone to wear and tear over time, which can lead to malfunctions if not replaced promptly. AI can track the usage and condition of these components, predicting their remaining lifespan.
Maintenance teams can use this information to schedule replacements before belts and chains fail, ensuring continuous operation.
7. Hydraulic System Failures
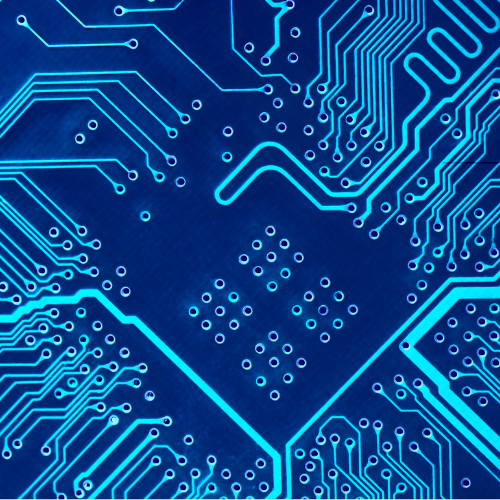
Hydraulic systems are essential for many industrial applications, but they are also prone to leaks and pressure loss. AI can monitor hydraulic pressure and fluid levels in real-time.
By analyzing these data points, AI can detect leaks or pressure drops early, enabling maintenance teams to address the issue before it escalates.
8. Pump and Valve Malfunctions
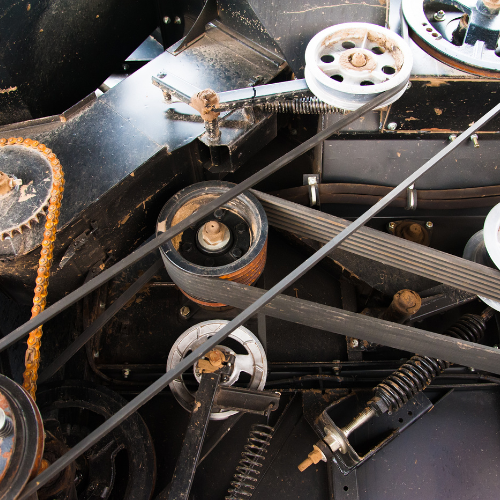
Pumps and valves play crucial roles in fluid and gas control systems. Malfunctions in these components can disrupt entire processes. AI can monitor the performance of pumps and valves, detecting signs of wear or malfunction.
By predicting failures, AI ensures that these components are maintained or replaced before they cause significant disruptions.
9. Gear and Shaft Misalignment
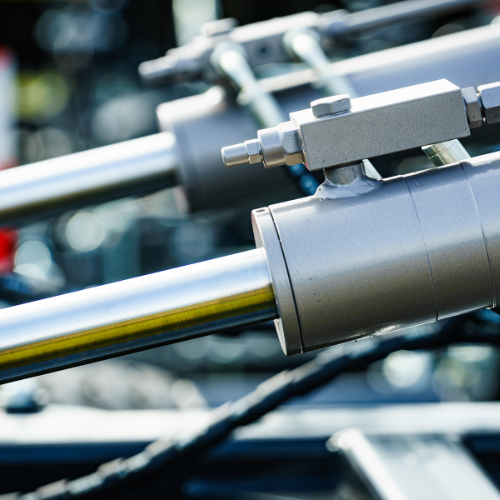
Misalignment of gears and shafts can lead to excessive wear and reduced efficiency. AI-powered sensors can detect misalignment by analyzing vibration and position data.
By identifying misalignment early, AI allows maintenance teams to realign components, preventing further damage and maintaining optimal performance.
10. Corrosion and Rust
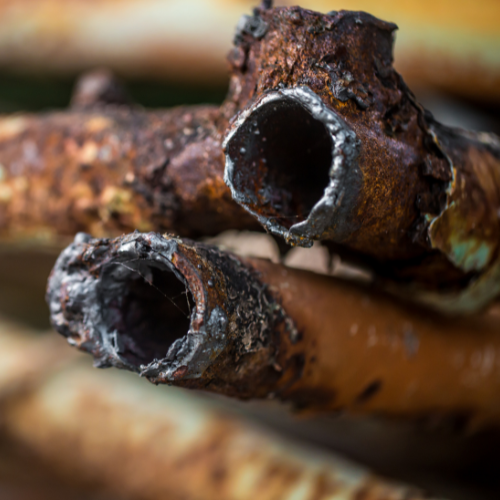
Corrosion and rust can compromise the integrity of machinery components, leading to failures. AI can monitor environmental conditions and material properties to predict the likelihood of corrosion.
By identifying areas at risk, AI enables preventive measures such as protective coatings or material replacements, extending the lifespan of equipment.
Case Studies: Success Stories of AI in Predictive Maintenance
Several companies have successfully implemented artificial intelligence in their smart factories to enhance predictive maintenance. For instance, a leading automotive manufacturer used AI to monitor and analyze data from assembly line robots.
By detecting early signs of wear and predicting failures, the company reduced downtime by 30% and increased production efficiency by 20%.
Similarly, an aerospace company implemented AI-driven predictive maintenance for their hydraulic systems, resulting in a 25% reduction in maintenance costs and improved system reliability.
Challenges and Solutions in Implementing AI for Predictive Maintenance
While the benefits of AI in predictive maintenance are clear, implementing these technologies can be challenging. Common challenges include:
1. Data Quality and Integration
- Ensuring that data from various sources is accurate and compatible can be difficult. To address this, companies can invest in robust data management systems and standardize data formats.
2. High Initial Costs
- Implementing AI and IoT technologies requires significant upfront investment. However, the long-term savings from reduced downtime and maintenance costs can justify the initial expenses.
3. Skills and Expertise
- Integrating AI into maintenance processes requires specialized skills and knowledge. Companies should invest in training programs and consider partnering with AI experts to build the necessary expertise.
4. Cybersecurity Concerns
- As smart factories become more connected, they are also more vulnerable to cyberattacks. Implementing strong cybersecurity measures, such as encryption and regular security audits, can help protect sensitive data and systems.
Future Trends in AI and Predictive Maintenance
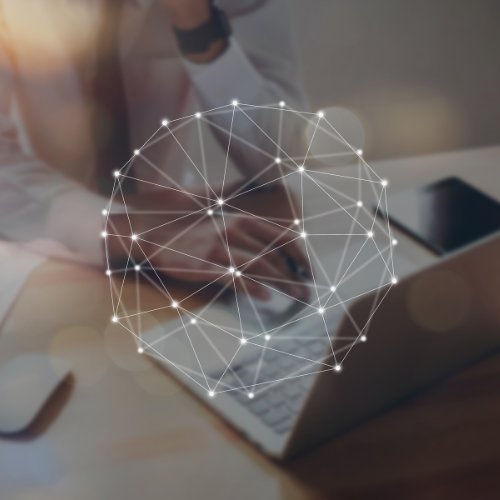
The future of predictive maintenance in smart factories looks promising, with several emerging trends set to enhance the role of artificial intelligence:
- Edge Computing
- Edge computing allows data to be processed closer to the source, reducing latency and improving real-time decision-making. This is particularly useful for predictive maintenance, where timely responses are crucial.
- Enhanced Machine Learning Algorithms
- Advances in machine learning algorithms will enable more accurate predictions and deeper insights into machinery performance. This will further reduce the likelihood of unexpected failures and optimize maintenance schedules.
- Integration with Augmented Reality (AR)
- Combining AI with AR can provide maintenance teams with real-time visualizations and instructions, improving efficiency and accuracy in performing maintenance tasks.
- Collaborative Robots (Cobots)
- Cobots equipped with AI can work alongside human workers, assisting in maintenance tasks and performing routine inspections. This collaboration can enhance productivity and safety in smart factories.
Conclusion
Artificial intelligence is transforming predictive maintenance in smart factories, preventing common machinery failures and optimizing production processes.
By leveraging AI to monitor and analyze data, smart factories can predict potential issues before they cause disruptions, reducing downtime and maintenance costs.
While there are challenges in implementing AI, the benefits far outweigh the initial investment. As technology continues to advance, the role of AI in predictive maintenance will only grow, making smart factories more efficient, reliable, and resilient.
In the era of digital transformation, artificial intelligence is not just a tool but a necessity for modern manufacturing. By understanding and embracing AI, businesses can ensure their operations remain competitive and future-proof.
Start integrating AI into your predictive maintenance strategies today and unlock the full potential of your smart factory.